Namita Dube, Ph.D. a staff scientist in the Montelione Lab made a significant contribution at the Annual Biophysical Society Meeting held February 9-15, 2024 in Philadelphia, PA. Namita showcased her expertise through the presentation of two impactful posters. Her work on “Structural Dynamics and Mechanisms of Antimicrobial Drug Transport by Integral Membrane Protein MipA using Deep Learning Methods and Anton-2 Simulations” included Figure 1 shown. As well as, “Flexibility and Stiffness in Interdomain Linkers of Gamma-Retrovirus Integrase Proteins” garnered widespread appreciation from scholars affiliated with prestigious universities.
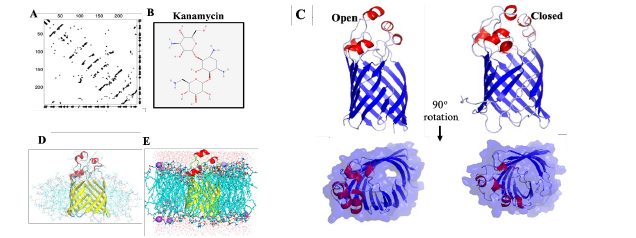
A: EC contacts (black circles). B. Structures of antibiotic used in this study.
C: AF2 predicted open and EC-NMR closed conformation of MipA. In
these contact maps, residue indices are plot on two axes, along with predicted secondary structure.
D,E: MipA open form in DPC detergent micelle, and POPE:POPG (3:1) bilayer.
Dr. Dube’s engagement and networking with fellow researchers at the conference brought valuable insights and perspectives to the ongoing studies in our laboratory. The meeting itself was a hub for innovative discussions on molecular dynamics and computational techniques, promising a treasure trove of methodologies and ideas beneficial for our research endeavors.
Dr. Dube’s presentations not only highlighted her contributions to the field but also underscored the dynamic, collaborative spirit of the Biophysical Society Meeting, enriching our lab’s knowledge base and inspiring future projects.
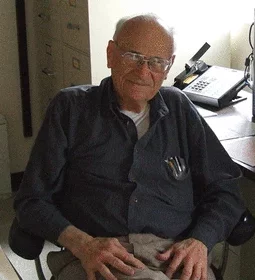
An Overview on the Occasion of Harold Scheraga’s 100th Birthday
Harold A. Scheraga presided over the advancement of the field of protein physical chemistry with magisterial vision and legendary intensity. From the earliest, precrystallographic efforts to determine protein structure hydrodynamically, through pathbreaking experiments on protein folding. To his most recent, cutting-edge work in computational protein chemistry and protein bioinformatics. He carried out seminal work on the elucidation of the blood clotting cascade and on the structure of water.
In addition to experimental work, he pioneered the fields of theoretical protein science, protein bioinformatics, computational structure prediction, and protein molecular dynamics. All current efforts in these fields rest on the shoulders of Scheraga’s early work.
His bibliography includes almost 1400 publications, he mentored over 400 graduate students and postdoctoral fellows, and he was honored with almost every award the field, and the chemical community at large, had to offer. He was truly a giant of science. Full text article featured in the virtual special issue of Journal of Physical Chemistry B (April 6, 2023).
Anton-2 supercomputer time by the National Research Council of the National Academy of Science (November 2022)
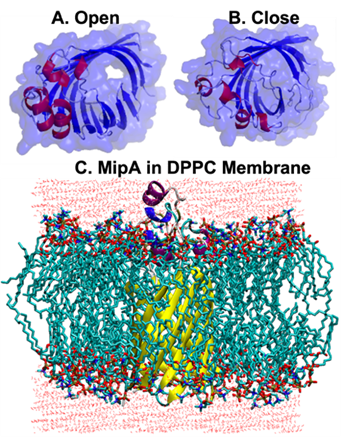
RPI Researchers awarded Anton-2 supercomputer time by the National Research Council of the National Academy of Science for investigating structural dynamics of membrane proteins that underpin antibiotic resistance.
The goal is to investigate mechanisms of antimicrobial drug transport by Integral Membrane Protein MltA Interacting Protein (MipA) using microsec-long molecular dynamic simulations.
To learn more about this research featured in RPI News click the link below. https://news.rpi.edu/approach/2022/11/21-0
National Institutes of Health Awards Grant for Enhanced NMR Instrumentation (January 2022)

Grant efforts were led by RPI Faculty Gaetano Montelione, Scott McCallum, Marimar Lopez, and Chunyu Wang Grants for 800 MHz NMR System Upgrade and Helium Recovery System. Article.
Hepatitis C drugs multiply effect of COVID-19 antiviral Remdesivir (April 2021)
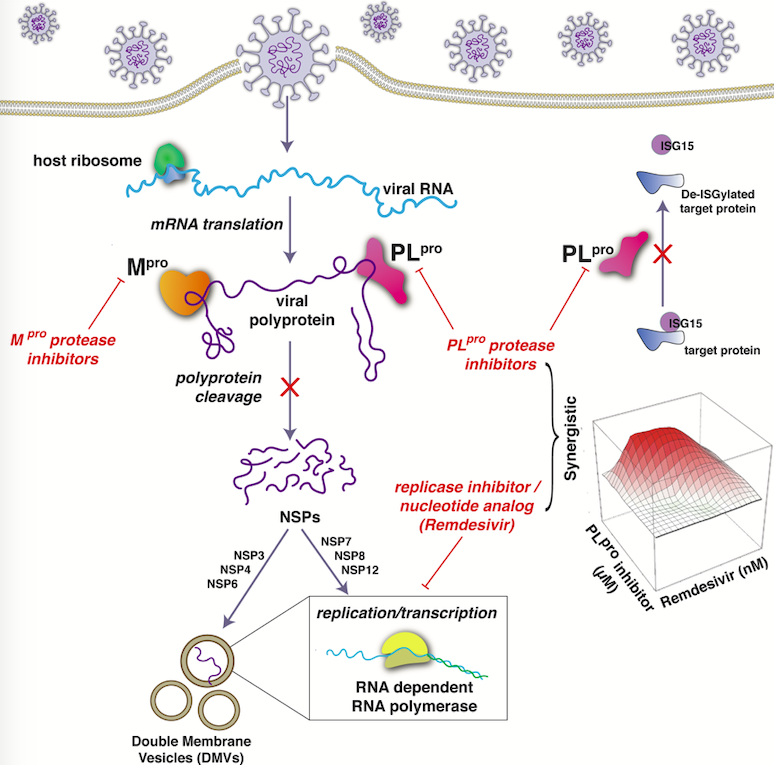
Proposed mechanism by which FDA-approved hepatitis C virus (HCV) drugs synergize with the SARS-CoV-2 antiviral remdesivir
Hepatitis C virus drugs inhibit SARS-CoV-2 PLpro and act synergistically with the antiviral remdesivir. Cell Reports 2021 Article; Press Release

Harold A. Scheraga
1921 – 2020
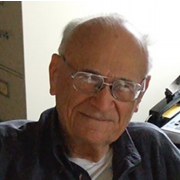
Harold was gifted with a unique combination of intensity and breadth of vision. He realized early in his career that both experimental and theoretical work would be necessary to solve the central problems of protein science, and, most unusually, he excelled in both spheres.
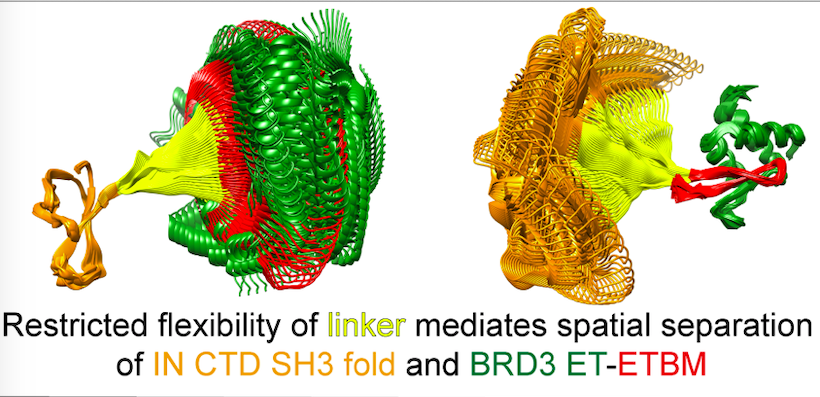
A common binding motif in the ET domain of BRD3 forms polymorphic structural interfaces with host and viral proteins
The extra-terminal (ET) domain of BRD3 is conserved among BET proteins (BRD2, BRD3, BRD4), interacting with multiple host and viral protein-protein networks. Solution NMR studies of complexes formed between BRD3-ET domain with either the 79-residue murine leukemia virus integrase (IN) C-terminal domain (IN329-408), or its 22-residue IN tail peptide (TP) (IN386-407) alone, reveal reveal a 10-residue linker region (IN379-388) tethering the SH3 domain (IN329-378) to the ET-binding motif (IN389-405)-ET complex. This linker has restricted flexibility, impacting the potential range of interdomain orientations in the IN – nucleosome complex.
Column 1
Image Box Title
Change this description
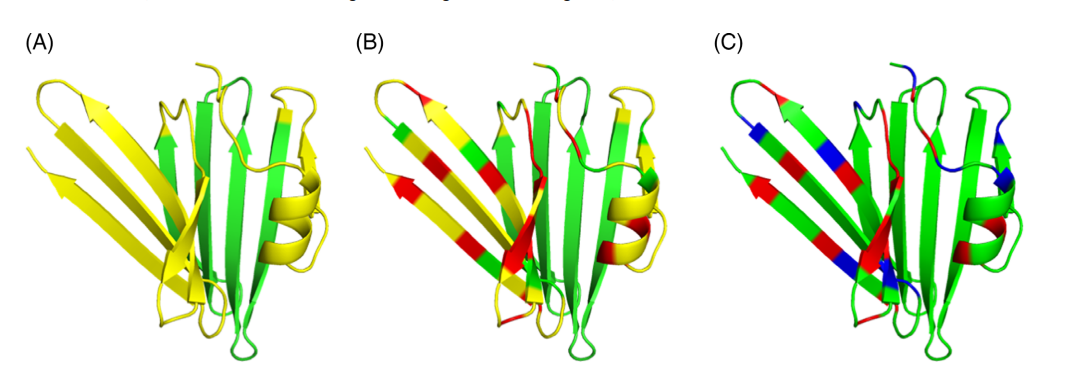
Schematic Depiction of process for simulating NOESY peak and resonance assignment data for CASP-NMR target N0968S1
Protein structure prediction assisted with sparse NMR data in CASP13
CASP13 has investigated the impact of sparse NMR data on the accuracy of protein structure prediction. NOESY and 15 N-1 H residual dipolar coupling data, typical of that obtained for 15 N,13 C-enriched, perdeuterated proteins up to about 40 kDa, were simulated for 11 CASP13 targets ranging in size from 80 to 326 residues. For several targets, two prediction groups generated models that are more accurate than those produced using baseline methods. Real NMR data collected for a de novo designed protein were also provided to predictors, including one data set in which only backbone resonance assignments were available. Some NMR-assisted prediction groups also did very well with these data. CASP13 also assessed whether incorporation of sparse NMR data improves the accuracy of protein structure prediction relative to nonassisted regular methods. In most cases, incorporation of sparse, noisy NMR data results in models with higher accuracy. The best NMR-assisted models were also compared with the best regular predictions of any CASP13 group for the same target. For six of 13 targets, the most accurate model provided by any NMR-assisted prediction group was more accurate than the most accurate model provided by any regular prediction group; however, for the remaining seven targets, one or more regular prediction method provided a more accurate model than even the best NMR-assisted model. These results suggest a novel approach for protein structure determination, in which advanced prediction methods are first used to generate structural models, and sparse NMR data is then used to validate and/or refine these models.